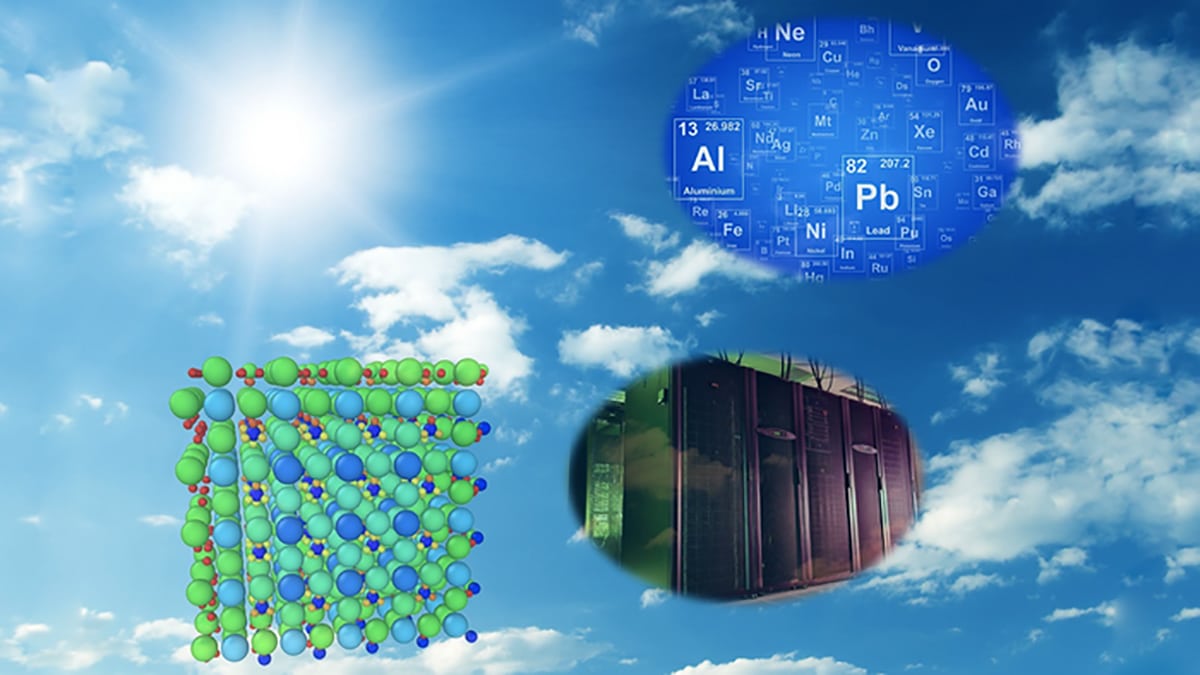
Solar absorbers are materials used to convert this energy into heat or electricity. A team of researchers at the U.S. Department of Energy’s (DOE) Argonne National Laboratory has developed a machine learning method for screening many thousands of compounds as solar absorbers.
A recent DOE study suggests that solar energy could power 40% of the nation’s electricity by 2035. According to the researchers, machine learning will play a vital role in realizing the lofty goal. A form of artificial intelligence (AI), machine learning uses a combination do large data sets and algorithms to imitate the way that humans learn. It learns from training with sample data and past experience to make even better predictions.
Currently, the primary absorber in solar cells is either silicon or cadmium telluride. But they remain fairly expensive and energy-intensive to manufacture. The researchers use their machine learning method to assess the solar energy properties of a class of material called halide perovskites.
Over the past decade, many researchers have been studying perovskites because of their remarkable efficiency in converting sunlight to electricity. They also offer the prospect of much lower cost and energy input for material preparation and cell building.
Argonne researchers trained their method with data for a few hundred halide perovskite compositions, then applied it to over 18,000 compositions as a test case. The method evaluated these compositions for key properties such as stability, ability to absorb sunlight, a structure that does not break easily due to defects, and more. They found that the computation agreed well with relevant data in the scientific literature. Also, the findings whittled down the number of compositions worthy of further study to about 400.
“Our list of candidates has compounds that have already been studied, compounds that no one has ever studied, and even compounds that were not among the original 18,000,” said Maria Chan, who led the research. “So we are very excited about that.”
The team next plans to test the predictions using experiments. The ideal scenario would be to use an autonomous discovery laboratory, such as Polybot at Argonne’s Center for Nanoscale Materials (CNM), a DOE Office of Science user facility. Polybot brings together the power of robotics with AI to drive scientific discovery with little or no human intervention.
By using autonomous experimentation to synthesize, characterize and test the best of their few hundred prime candidates, Chan and her team anticipate they can also improve the current machine learning method.
“We are truly in a new era of applying AI and high-performance computing to materials discovery,” said Chan. “Besides solar cells, our design methodology could apply to LEDs and infrared sensors.”
Artificial intelligence for extracting the sun’s energy
Source: Tambay News
0 Comments